Register to attend our ALS Town Hall on April 21 to hear ALS TDI's CEO, Fernando Vieira M.D. discuss this exciting research and answer questions from the community. Register here.
One of the most important goals of the ALS Therapy Development Institute’s (ALS TDI) Precision Medicine Program (PMP) since its inception has been to discover objective measures of Amyotrophic Lateral Sclerosis (ALS) progression. In late April, ALS TDI and our collaborators at Google made an important step toward making this goal a reality for people living with ALS with the publication of a paper describing their research to create artificial intelligence tools for scoring ALS-related symptom severity.
Creating an Objective Measure for ALS Symptom Severity
The paper, titled A Machine-Learning Based Objective Measure for ALS Disease Severity, details how data from the PMP was utilized by ALS TDI scientists and Google AI researchers to develop a machine learning algorithm that can analyze voice recordings and accelerometer movement data. This algorithm is able to look at these data and assign scores corresponding to an individual’s speech and movement abilities, potentially allowing them to accurately and, importantly, objectively track changes in these abilities over time.
For years, the ALS Functional Rating Scale (ALSFRS-r) has been the main tool for clinicians and researchers to measure ALS disease progression. Although it is the current standard, the ALSFRS-r is limited by its subjectivity – it relies on either a clinician or subject’s self-reported observations of how well they can move, speak, and perform other functions. This machine learning algorithm, on the other hand, can provide an objective numerical score that should be internally consistent throughout a person’s disease progression.
The Critical Role of AI and PMP Participant Data
This is possible because of the combination of Google’s expertise in artificial intelligence and machine learning with the wealth of data provided by participants in ALS TDI’s PMP. To develop the algorithm, the paper’s authors utilized thousands of voice recordings, accelerometer samples, and years self-reported ALSFRS-r scores from 584 people living with ALS. The results are two algorithms that can predict a person’s ALSFRS-r score in the movement and speech ALSFRS-r categories with a strong correlation to their self-reported scores.
Sharing Findings with the Community
The paper is still undergoing peer review, and will be hopefully be published in a peer-reviewed scientific journal later this year. However, the authors chose to make it available publicly early on medRXiv.org, an online repository for preprint publications in the health sciences. Additionally, they have posted the code for the algorithms on GitHub, a development platform that will allow anyone to access it, use it, and, potentially, even make changes to improve upon it.
Today, scores automatically generated by the algorithm are available to participants in the PMP through their personalized online portal. However, the unmet need for objective measures of ALS progression goes far beyond the participants in the program. Thus, the authors chose to make the paper and code available to any researchers or clinicians who might hope to use it as soon as possible
Their hope is that by disseminating this research as widely as possible that others in the ALS space might take advantage of the algorithm as it exists now to track disease progression in clinical and research settings. Additionally, ALS TDI researchers look forward to improvements to the algorithm itself that may come from others working with it – or new applications of machine learning techniques in ALS that might be inspired by it. The goal is, ultimately, to spur the further development of reliable and objective digital measures for ALS progression that will aid clinicians and make clinical trial data more efficient and accurate.
What to do next
- Read the paper, A Machine-Learning Based Objective Measure for ALS Disease Severity, here.
- Check out the code for the machine learning algorithm on GitHub.
- Learn more and enroll in ALS TDI’s Precision Medicine Program.
Key takeaways
- ALS TDI and Google researchers have shared a study about a machine learning algorithm they have developed for scoring ALS-related changes in speech and movement ability.
- This algorithm was developed with data provided over several years by participants in ALS TDI’s precision medicine program.
- Developing objective measures such as this is important because the main tool we have now for tracking ALS-related functional decline – the ALSFRS-r – is based on subjective observations that can vary from person to person.
- The paper’s authors decided to share it to encourage other researchers and clinicians to use this tool – and potentially to improve upon it. They also made the code for the algorithm publicly available.
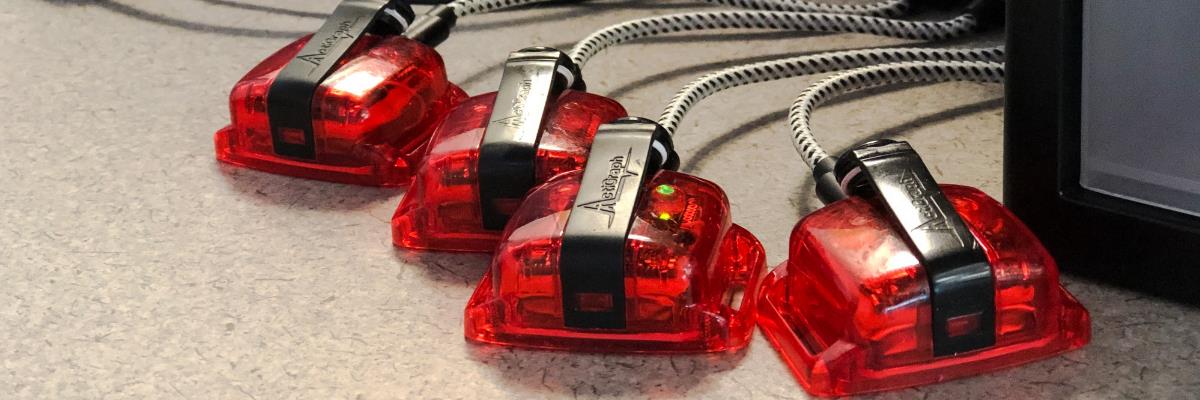